Atmospheric physics-guided machine learning for climate modeling and weather forecasting
Data-driven algorithms, in particular neural networks, can (1) emulate the effect of unresolved processes in coarse-resolution climate models if trained on high-resolution simulation data; and (2) significantly improve the skill of numerical weather forecasts at low computational cost if trained on observations. However, they may violate key physical constraints and make large errors when evaluated outside of their training set. I will share progress towards overcoming these two challenges in the case of machine learning (1) the effect of subgrid-scale convection and clouds on the large-scale climate; and (2) the bias correction of near-surface temperature and humidity to post-process numerical weather predictions.
First, physical constraints can be enforced in neural networks, either approximately by adapting the loss function or to within machine precision by adapting the architecture. Second, as these physical constraints are insufficient to guarantee generalizability, we additionally propose to transform the inputs and outputs of machine learning algorithms using established physical rescalings to help them generalize to unseen climates and locations. Overall, these results suggest that explicitly incorporating physical knowledge into data-driven models for weather and climate applications may improve their consistency, stability, and ability to generalize across atmospheric regimes.
Data-driven algorithms, in particular neural networks, can (1) emulate the effect of unresolved processes in coarse-resolution climate models if trained on high-resolution simulation data; and (2) significantly improve the skill of numerical weather forecasts at low computational cost if trained on observations. However, they may violate key physical constraints and make large errors when evaluated outside of their training set. I will share progress towards overcoming these two challenges in the case of machine learning (1) the effect of subgrid-scale convection and clouds on the large-scale climate; and (2) the bias correction of near-surface temperature and humidity to post-process numerical weather predictions.
First, physical constraints can be enforced in neural networks, either approximately by adapting the loss function or to within machine precision by adapting the architecture. Second, as these physical constraints are insufficient to guarantee generalizability, we additionally propose to transform the inputs and outputs of machine learning algorithms using established physical rescalings to help them generalize to unseen climates and locations. Overall, these results suggest that explicitly incorporating physical knowledge into data-driven models for weather and climate applications may improve their consistency, stability, and ability to generalize across atmospheric regimes.
Co-Authors (in alphabetical order): Pierre Gentine (Columbia University), Daniele Nerini (MeteoSwiss), Michael Pritchard (UCI, NVIDIA), Stephan Rasp (Google Research), Francesco Zanetta (ETH, MeteoSwiss)
Speakers
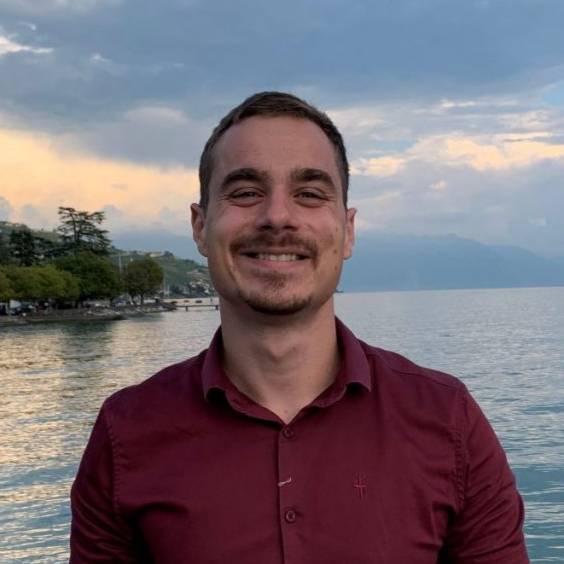
Tom Beucler is an assistant professor of environmental data science at the University of Lausanne in Switzerland. He recently started a lab (https://unil.ch/dawn) specifically dedicated to the intersection of atmospheric physics and machine learning, with the goal of improving our understanding of atmospheric dynamics and assisting weather and climate predictions. For that purpose, his research group combines physical theory, computational science, statistics, numerical simulations, and observational analyses. Before that, Tom studied the interaction of tropical storms, radiation, and atmospheric water as part of his PhD at MIT. As a postdoc and project scientist at Columbia and UC Irvine, he investigated how to best integrate physical knowledge into neural-network representations of convection for climate modeling.