Counterfactual inference made easy
Counterfactuals are hypothetical statements that we continuously use throughout the day, e.g., would I have gotten home earlier had I taken the cantonal road rather than the freeway? We use them to reflect on our behaviour and improve it in the future, through mechanisms such as regret and credit. But also for legal reasoning, fairness, ethics, and to properly evaluate the impact of policies. Having computers handle counterfactuals can be pursued using Pearl’s structural causal models. These are collections of structural equations that typically involve latent terms, and which often lead to unidentifiable problems. In this talk, we shall see that general counterfactuals can easily be computed for categorical variables even when the only information available is a causal graph among them plus data about its manifest nodes.This is achieved by an EM-based approximate algorithm along with guarantees computed via credible intervals. The algorithm is then extended to smoothly handle selection bias as well as a complex setting of information fusion with multiple data sources of multiple types (observational or interventional). Experiments and a real-world problem in palliative care will exemplify the application of our method.
Counterfactuals are hypothetical statements that we continuously use throughout the day, e.g., would I have gotten home earlier had I taken the cantonal road rather than the freeway? We use them to reflect on our behaviour and improve it in the future, through mechanisms such as regret and credit. But also for legal reasoning, fairness, ethics, and to properly evaluate the impact of policies. Having computers handle counterfactuals can be pursued using Pearl’s structural causal models. These are collections of structural equations that typically involve latent terms, and which often lead to unidentifiable problems. In this talk, we shall see that general counterfactuals can easily be computed for categorical variables even when the only information available is a causal graph among them plus data about its manifest nodes.This is achieved by an EM-based approximate algorithm along with guarantees computed via credible intervals. The algorithm is then extended to smoothly handle selection bias as well as a complex setting of information fusion with multiple data sources of multiple types (observational or interventional). Experiments and a real-world problem in palliative care will exemplify the application of our method.
Speakers
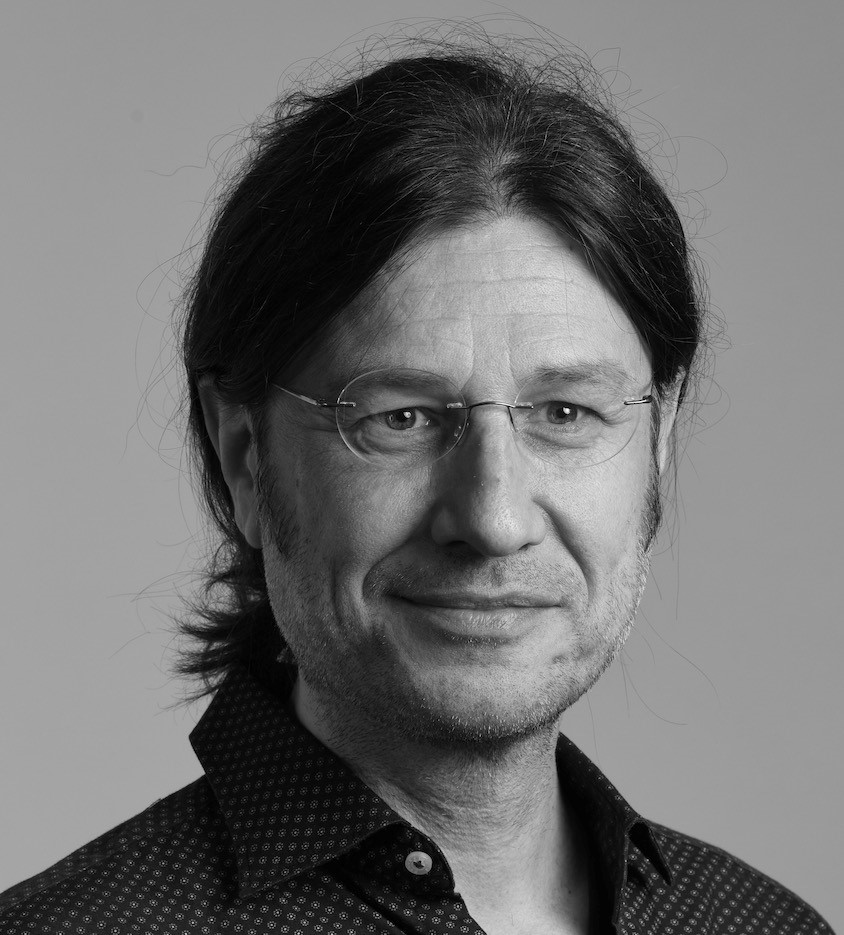
Marco Zaffalon is a University Professor and Scientific Director at IDSIA. He has 160+ refereed publications on artificial intelligence and machine learning, and his research has overall been supported by 15 million francs in competitive grants. Marco is a Senior Area Editor of the International Journal of Approximate Reasoning, as well as Senior Program Committee Member for IJCAI, AAAI, and UAI, and has been a past President of the Society for Imprecise Probability.At IDSIA he founded the group on probabilistic machine learning that has grown up to 40 researchers. In his applied research he has worked with UBS, Novartis, Mastercard, and several other world’s largest companies.In 2020 he co-founded Artificialy, a company for products and services in AI employing 25 people, where he works as the company's Chief Scientist.